DATA INFRASTRUCTURES
How the Rise of Generative AI is Impacting Data Centers and Network Infrastructures
Overview
Although AI has been around in the form of machine learning and data analytics applications used behind the scenes by many enterprises for years, the recent rise of Generative AI applications that respond to natural language inquiries from users is taking AI into exciting new arenas.
As these new applications are moving AI into the mainstream, the need for real-time, low-latency processing of Large Language Model (LLM) databases to provide on-the-fly responsiveness is having major impacts on data centers and networks. This means that existing data and communications infrastructures must undergo radical transformation and expansion to meet the new AI demands.
This article provides a forward look into the impact of new demanding generative AI applications on data centers, with a focus on the changes that will be necessary to support new requirements for scaling up massive computing and delivering low-latency responsiveness.

Trends in New AI Use Cases

Generative AI: Generative AI involves the creation of new data, such as images, text, music, or video, by AI models. This technology has applications in various fields, including content creation, design, gaming, and virtual reality. Generative adversarial networks (GANs), that are designed for iterative self-correction learning, have shown remarkable progress in generating realistic, high-quality content.

AI in Natural Language Processing (NLP): NLP continues to evolve, with advancements in language understanding, sentiment analysis, and language generation. OpenAI's GPT models, for instance, have demonstrated impressive language generation capabilities. Future applications include more natural and conversational virtual assistants, improved language translation, and enhanced content creation.

Edge AI: Edge computing combined with AI is gaining traction. By deploying AI algorithms and models directly on edge devices, such as smartphones, IoT devices, and autonomous vehicles, real-time decision-making and local data processing can be achieved. This enables faster response times, reduced latency, and improved privacy.

Explainable AI (XAI): Explainable AI focuses on making AI models and their decisions transparent and interpretable to humans. It aims to address the "black box" nature of deep learning models and provides insights into why certain decisions or predictions are made. XAI is crucial for building trust in AI systems, especially in domains like healthcare, finance, and law.

AI in Robotics and Automation (XAI): The integration of AI with robotics is advancing automation capabilities across industries. Collaborative robots, or cobots, equipped with AI can work alongside humans in manufacturing and assembly tasks. AI also enables robots to learn and adapt to new environments, enhancing their autonomy and versatility.

AI for Cybersecurity: As cyber threats become more sophisticated, AI is being employed to strengthen cybersecurity measures. AI algorithms can detect anomalies in network traffic, identify patterns of malicious activity, and prevent cyber-attacks. AI-driven cybersecurity systems can respond and adapt to evolving threats in real-time, providing enhanced protection.

AI in Personalized Medicine: AI is revolutionizing healthcare by enabling personalized medicine approaches. Machine learning models can analyze large-scale patient data, genetic information, and medical records to identify patterns and correlations. This can aid in disease diagnosis, treatment selection, and predicting patient outcomes, leading to more effective healthcare interventions.

AI for Climate Change and Sustainability: AI is being explored to address pressing environmental challenges. It can help analyze climate data, optimize energy consumption, predict weather patterns, and develop sustainable solutions. AI-powered systems have the potential to optimize resource utilization, reduce emissions, and contribute to environmental conservation.
AI Impacts on Data Centers and Networks



Overall, the rise of AI will drive the demand for more powerful hardware, increased network bandwidth, the development of edge computing capabilities, and higher power usage. It will also make possible AI-enabled improvements in network optimization, automation, and enhanced security measures to support the growing demands of AI applications.
Tech Innovations to Support Infrastructure Improvements for AI
- Interconnects (higher density, robust, automation-friendly)
- Connectors (solder-free, customized, modular)
- Enclosures (power sources, cooling and thermal solutions, rackmount)
- Module-level and vertical integration
Key enabling technologies include Press-Fit interconnects, customizable busbars, SSD carriers, HDD carriers, heatsinks, network enclosures and custom design application-specific vertical integration solutions.

Summary
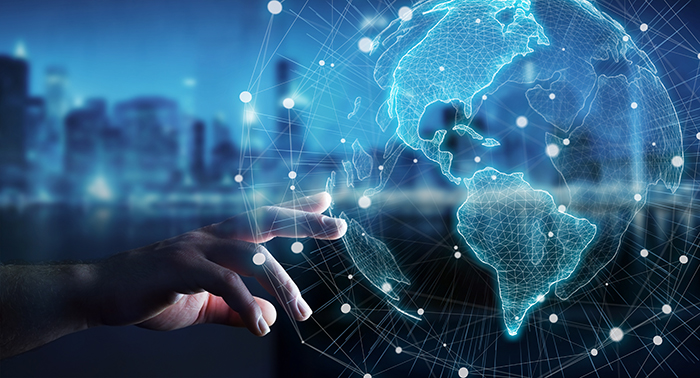
At Interplex, we are proud and excited to play a key role in helping to innovate designs and deliver targeted solutions all up and down the technology stack that will fuel the sweeping changes needed to make the promise of new AI capabilities a reality for companies and consumers everywhere in the world.
With our manufacturing facilities in diverse locations, customers can tap into our regional value chains to reduce time-to-market and transportation costs and improve response time to changes.